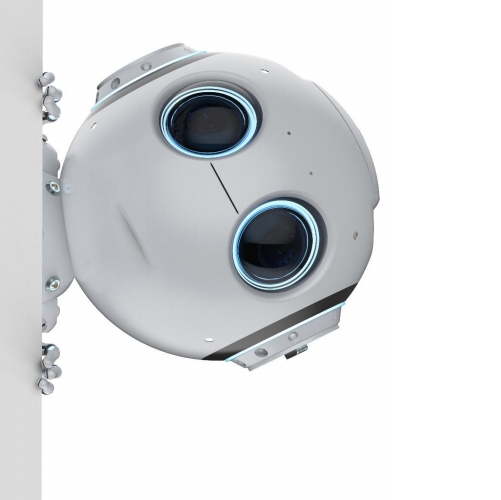
The Gartner agency is predicting the hype of KGs and conversational AI. The main drivers are sales services, customer support, and lower development costs for a chatbot. Like with all crafts, mastery of graph thinking, and understanding gained through continued practice, since its inception by Google, Knowledge Graph has come to be a newly universally used period so far does not have a well-recognized explanation. Remember, there are many state-of-the-art graphs: Freebase, Google KG and Knowledge Vault, Cyc and Open Cyc, WikiData, DBpedia, YAGO, NELL, Microsoft Satori KG. In this chapter, we discovered the basics of graph market, algorithms, and schemas. We also extracted data from the real dataset and produced our graph from scratch. In the next chapter, we will cover a detailed overview of knowledge graphs in the industry. We will uncover details and techniques on automatic graph building using natural language techniques like inference and cognitive toolkits. Machine learning and knowledge graphs (KG) are linked together with Natural language processing (NLP).
Ontology in different data science fields
When we connect ontologies, schemas to data science, it is indeed a fruitful discussion. What is text mining with NLP or data analytics if schema or ontology is not well-defined? It is impossible to do the most basic text mining, or coherent navigation, or filtering. Knowledge about the world is absorbed from books, articles, and papers on the web. The data is noisy and self-contradictory. Most of the time, in the first place, when analyzed, but when ingested with all rules and languages like SPARQL and semantically aligned, it is useful. Let's say you have a list of documents on your Google Drive or Dropbox, and you want to link them with pictures, databases, or other forms. It is probably easier to structure them by special schemes, extension types of the document, and semantics inside them. What will be the top entities in each of them, and why could we connect them to specific ontology relations. If you think about Wikipedia as an example, there are 200 people with Will Smith's name. If you dive a bit further from Wiki, there are 41 sites with more famous actors' information.
When we were attacking a bit further and extract triples or facts about the entity, there are 108,000. The vital skill to learn is how to absorb all this knowledge with SPARQL and other semantic languages and leverage it later for experiences and applications. Empowering the system's automated reasoning is challenging when you go beyond simple queries or searches for lookup.
Semi-Structured data without semantics
There is a benefit of ontology because data is structured to help understand the semantics, and relationships between entities are more transparent. Wikidata has 52 million entities in it and 3.9 billion facts and stored on triples. Graph databases provide a way to see the insights and reasoning on complex data. Sometimes, the ingestion data contradicts the existing infrastructure and schemas available in a triple store and needs to be modified. The ingestion process uncovers similarities, groups, and patterns that were not visible before. It is criticial to have quality gates and golden set tests to validate if you are not breaking existing pipelines while creating or ingesting new entities. Making data machine-readable is the most challenging problem we phase every day, but it makes our lives so much easier as humans when it is done and working.
Semantically Linked Graph Data
Get a definition of ontology for the COVID Kaggle data set
As I promised to you initially, it is crucial to have some exercise in understanding the concepts described in the chapter on the real example of a graph cloud database. We will cover Tiger Graph architecture and real-life examples based on the COVID-19 data sets from 2020. The data set itself is not much used for the industry anymore because it is old, but it is more about the approach and how we could do it step by step together.
Please look at the White House research data set about the pandemic and a description of what it has. Most of the Open Research Dataset (CORD-19) data, but 200K articles with 100K full-text articles on SARS-CoV-2 and other coronaviruses. Pandemic forces everyone to share data, approve reports faster, and recent NLP advances allow us to use AI to get more insights about ongoing infectious disease.
It is crucial to do rapid acceleration of work and research, so I decided to include it. You could start using Tiger Graph for free, and it was easy to set up, so please try it yourself. As far as I like it, the most significant features are usability and a user-friendly interface for visualizing the graph query language, deep link analytics, and how we could scale faster.
The Tiger Graph was an excellent way to show you techniques for leveraging ontology and building schemas for entities in the data set by hand. Let us create an easy and free Tiger Graph demo together. Here is the URL: https://tgcloud.io
Tiger graph cloud database
We will have to sign up and create a new account in the graph. Once you sign up, you could select a free instance of the cloud. We are going to use an hourly free service. Go to My Solutions and Create Solutions. You could experiment with fraud, healthcare, blank, or knowledge graphs. For now, we could select a starter kit with AWS Free tier where we can get 7 GB memory. Right away, let us start to look at the data and clean up and optimize it a bit. Creation could take 5-10 minutes, and provision will take a bit more in the Azure or AWS cloud. Here is the Kaggle challenge related to this open-source data set: https://www.kaggle.com/allen-institute-for-ai/CORD-19-research-challenge.
Check out tutorials on how to run the Kaggle graph data instance, for example, scispaCy. scispaCy is a Python package including spaCy models for handling biomedical or clinical text. https://github.com/akash-kaul/Using-scispaCy-for-Named-Entity-Recognition When you look at the real COVID-19 ontology examples, you will find that we must define it for each column in the data and each class like Entity, Publication, Author, License, etc. After we have them as vertex types, we could see relationships:
Example of Tiger Graph Solution
The exercises below will teach us how to build a schema and ontology yourself. It will allow you to validate the data on ingestion and load a real data set from the White House. The full-stack hosts on the scalable AWS (amazon cloud) instance that is free to use in the settings
Tiger Graph Solution Creation with the free option
Graph Studio Data Schema
Dataset has journals and entities connected to the author's publications. Suppose you want to see what we could do next after all training and entity linking done, and our result might look like after all such hassles. In that case, you could find the following distant and weak supervision on the named entity recognition (NER) for COVID-19 data: https://arxiv.org/pdf/2003.12218.pdf Below is the example of how tagged NER data.
COVID-19 data set data exploration. The tiger graph allows using a friendly user interface for filtering the graph data by type. You could see the color highlights of entities and easily explore the new data set with queries.
Q&A
- How do we think about the data as a graph, and how will we model a schema?
- Does your problem need graph data to answer complex questions?
- What is the difference between Amazon, Facebook, LinkedIn, Microsoft, and Google graphs?
- Why is Freebase graph data usage not recommended? Think about how it refreshed?
- What correctly supports assistants, chatbots, and search engines in the backend?
- Why do we need graph embeddings, and how are they different from word embeddings?
- What are you going to do through the relationships in your data?
- How to manage operations on the graph with scale and structured/unstructured sources?
- How to do management for changing knowledge? How do inference and verification?
- How entity disambiguation and linking are helping to manage identity uniquely?
- What to do with global, domain-specific, and customer-specific knowledge?
- How to organize multilingual and multidimensional systems for graph knowledge representation?
Further Reading