As of 2012, about half of all adults, 117 million people have one or more chronic diseases. One of four adults has two or more chronic diseases (CDC) 84% of all health care spending in 2006 was for the 50% of the population who have one or more chronic health conditions. By 2020 the number of people (adults and children) with chronic conditions is projected to be 157 million people. By 2020, medical information to double every 73 By 2018, 40% of primary care encounters in the U.S. will be delivered virtually. Medical data is expected to double every 73 days by 2020. Also 80% of health data is invisible to current systems because it's unstructured. The measure of a patient's overall health goes beyond medical and genomic conditions. In fact, almost 75% of a patient's status is affected by a host of lifestyle factors like access to shelter, education, income...
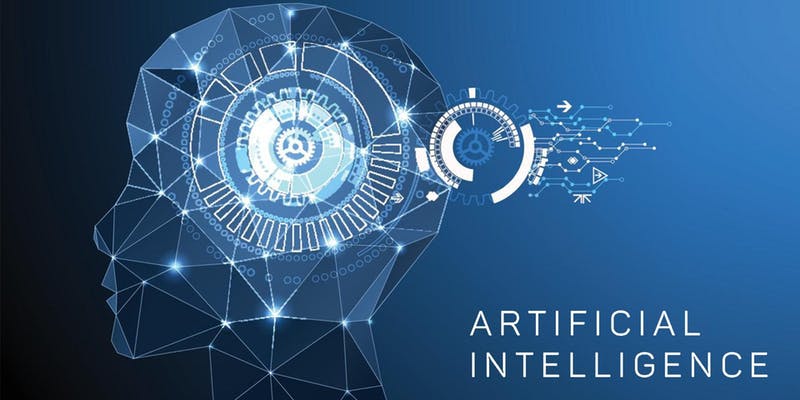
Analysis of medical images is essential in modern medicine. With the ever-increasing amount of patient data, new challenges and opportunities arise for different phases of the clinical routine, such as diagnosis, treatment, and monitoring. The Inner Eye research project focuses on the automatic analysis of patients' medical scans. It uses state-of-the-art machine learning techniques for the:
•Automatic delineation and measurement of healthy anatomy and anomalies;
Robust registration for monitoring disease progression;
•Semantic navigation and visualization for improved clinical workflow;
•Development of natural user interfaces for medical practitioners.
The introduction of cloud computing and machine learning is helping researchers identify ways to reduce hospital readmissions for chronic conditions and provide corresponding actionable guidelines for patient-provider teams.
Researchers in machine learning - including those working in statistical natural language processing, computer vision and related sub-fields when coupled with seasoned clinicians can play an important role in turning complex medical data (e.g., individual patient health records, genomic data, data from wearable health monitors, online reviews of physicians, medical imagery, etc.) into actionable knowledge that ultimately improves patient care. For the last six years, MUCMD has drawn about 100 clinical and machine learning researchers to frame problems clinicians need solved and discuss machine learning solutions.
Dossia is a Personal health record service offered by some of the largest employers in the United States. Dossia differs from traditional tethered PHR services by providing user access to health information regardless of health plan, employer, or physician. Users also have the ability to download their full record, in electronic form, at any time.
Microsoft HealthVault is a web-based personal health record created by Microsoft, in October 2007, to store and maintain health and fitness information. This website addresses both individuals and healthcare professionals, and in June 2010 expanded its services beyond the United States to include the United Kingdom.
Google's DeepMind health-care application on Bloomberg West. Deepmind creates health-care app. Statement of intent from Google to compete with IBM.
Google has filed U.S. Patent Application #20070282632, "Method and apparatus for serving advertisements in an electronic medical record system"
Google Health has been permanently discontinued. All data remaining in Google Health user accounts as of January 2, 2013 has been systematically destroyed, and Google is no longer able to recover any Google Health data for any user. To learn more about this announcement, see our blog post, or answers to frequently-asked questions below. When we launched Google Health, our goal was to create a service that would give people access to their personal health and wellness information. ... with a few years of experience, we've observed that Google Health is not having the broad impact that we hoped it would.
We need tools that can help us – both clinicians and patients – make better healthcare decisions. Yet in order to do so, those tools need to think like we do … and the key to natural intelligence is not simply X better than Y, but rather sequences of decisions over time. To best assist us, our clinical computing tools should approximate the same process. Such an approach ties to future developments across the broader healthcare space: cognitive computing, smart homes, cyborg clinicians, and robotics.
1. Disease Identification and Diagnosis:
- ML algorithms can analyze complex medical data and assist in diagnosing diseases, sometimes even before symptoms appear. For example, machine learning models are used to analyze images like X-rays, MRIs, and CT scans to identify cancerous tumors, fractures, and other abnormalities with high accuracy.
2. Drug Discovery and Development:
- ML speeds up the process of drug discovery by predicting how different chemicals will react with each other and identifying potential candidates for pharmaceuticals faster than traditional laboratory experiments. This can significantly reduce the time and cost associated with bringing new drugs to market.
3. Personalized Medicine:
- ML algorithms can analyze data from various sources, including genetic information, to tailor treatments to individual patients. This personalized approach improves the effectiveness of treatments and reduces side effects.
4. Predictive Analytics for Patient Monitoring:
- Machine learning models can predict patient outcomes based on historical data, allowing healthcare providers to intervene earlier and prevent hospital readmissions or other adverse events. Wearable devices and sensors contribute data that ML algorithms analyze to monitor chronic conditions or detect emergent health issues.
5. Epidemiology and Public Health:
- ML helps in tracking and predicting disease outbreaks by analyzing data from various sources, including social media, mobility data, and healthcare records. This assists in public health planning and response strategies.
6. Operational Efficiency:
- Healthcare facilities use ML to optimize scheduling, reduce wait times, manage patient flow, and improve service delivery, leading to better patient care and reduced costs.
7. Medical Research and Clinical Trials:
- ML algorithms analyze vast amounts of data from clinical trials to identify patterns and insights, speeding up research and improving the accuracy of findings. They can also help in patient selection for clinical trials, ensuring that the trial population accurately represents the patient demographics.
8. Mental Health:
- Machine learning models are being developed to detect signs of depression and anxiety through speech and text analysis. They can also provide personalized recommendations for therapy or interventions based on patient data.
9. Robotic Surgery and Assistive Technologies:
- ML enhances the capabilities of robotic surgical systems, improving precision and reducing human error. Assistive technologies powered by ML can help individuals with disabilities or chronic conditions to lead more independent lives.
Challenges and Considerations:
While the integration of ML in healthcare offers immense potential, it also poses challenges, including data privacy concerns, the need for large, diverse datasets to train algorithms, and the importance of interpretability and transparency in ML models. Ensuring equity in healthcare outcomes and addressing biases in ML algorithms are critical considerations as these technologies become more prevalent.
Overall, machine learning is becoming an integral part of the healthcare landscape, offering solutions to longstanding challenges and opening new avenues for treatment and research. However, successful implementation requires careful consideration of ethical, legal, and social implications.
References:
https://www.ibm.com/watson/health/
http://healthcareconferencenetwork.com/machine-learning-healthcare/
http://www.healthcareitnews.com/news/artificial-intelligence-cognitive-computing-and-machine-learning-are-coming-healthcare-it-time
https://en.wikipedia.org/wiki/Google_Health
https://en.wikipedia.org/wiki/Microsoft_HealthVault
https://en.wikipedia.org/wiki/Dossia
http://mucmd.org/
http://www.cognitivescale.com/how-cognitive-computing-and-ai-can-help-us-healthcare-companies-thrive-amidst-disruption/#sthash.pt7S90ma.dpuf
Summary
Medical errors remain a significant problem in healthcare. Electronic medical records (EMRs) have shown great promise in helping health care providers to identify and reduce medical errors. Computer-based monitoring and alerting systems play a key role in this effort. Distributed network architecture and data analytics have in many ways revolutionized the conduct of multi-center, population-based research of medical products. Anomaly detection in healthcare data is an enabling technology for the detection of over payment and fraud. Massive amounts of medical data from electronic health records and body-worn sensors are being mined by researchers, and could be used to guide the decision-making of both doctors and patients. The use of databases of routinely collected healthcare information in epidemiology has expanded in the last decade as awareness has increased and more and larger resources have become available. However, health care lags behind other industries in leveraging advances in information technology and analytical techniques. Achieving the dual goal of improving health outcomes and controlling cost will require making better decisions for the individual. Relying on traditional randomized control studies will be insufficient to promote personalized healthcare. Analysis of real world data, big data, in all its forms, will be necessary. Reducing avoidable healthcare encounters, in which home monitoring has a role, is one improvement strategy.