A good understanding of big-O analysis is critical to making a good impression with the interviewer. Big-O analysis is a form of run-time analysis that measures the efficiency of an algorithm in terms of the time it takes for the algorithm to run as a function of the input size. It’s not a formal benchmark, just a simple way to classify algorithms by relative efficiency.
In mathematics, computer science, and related fields, big-O notation (also known as big Oh notation, big Omicron notation, Landau notation, Bachmann–Landau notation, and asymptotic notation) (along with the closely related big-Omega notation, big-Theta notation, and little o notation) describes the limiting behavior of a function when the argument tends towards a particular value or infinity, usually in terms of simpler functions. Big O notation characterizes functions according to their growth rates: different functions with the same growth rate may be represented using the same O notation.
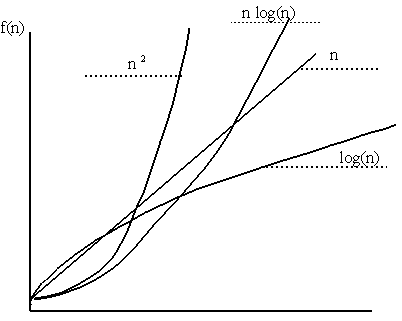
How to Do Big-O Analysis
The general procedure for big-O run-time analysis is as follows:
- Figure out what the input is and what n represents.
- Express the number of operations the algorithm performs in terms of n.
- Eliminate all but the highest-order terms.
- Remove all constant factors.
For the algorithms you’ll be tested on, big-O analysis should be straightforward as long as you correctly identify the operations that are dependent on the input size.
Most coding problem solutions include a run-time analysis to help you solidify your understanding of the algorithms.
Analyzing Two Examples
Let’s start with an example of big-O analysis in action. Consider a simple function that returns the maximum value stored in an array of non-negative numbers. The size of the array is n. There are at least two easy ways to implement the function. In the first alternative, you keep track of the current largest number as the function iterates through the array and return that value when you are done iterating. This implementation, called CompareToMax, looks like this:
The second alternative compares each value to all the other values. If all other values are less than or equal to a given value, that value must be the maximum value. This implementation, called CompareToAll, looks like this:
Both of these functions correctly return the maximum value. Which one is more efficient? You could try benchmarking them, but it’s usually impractical (and inefficient) to implement and benchmark every possible alternative. You need to be able to predict an algorithm’s performance without having to implement it. Big-O analysis enables you to do exactly that: compare the predicted relative performance of different algorithms.
How Big-O Analysis Works
In big-O analysis, input size is assumed to be n. In this case, n simply represents the number of elements in an array. In other problems, n may represent the number of nodes in a linked list, or the number of bits in a data type, or the number of entries in a hash table, and so on. After figuring out what n means in terms of the input, you have to determine how many times the n input items are examined in terms of n. “Examined” is a fuzzy word because algorithms differ greatly. Commonly, an examination might be something like adding an input value to a constant, creating a new input item, or deleting an input value. In big-O analysis, these operations are all considered equivalent. In both CompareToMax and CompareToAll, “examine” means comparing an array value to another value.
In CompareToMax, each array element was compared once to a maximum value. Thus, the n input items are each examined once, resulting in n examinations. This is considered O(n), usually referred to as linear time: The time required to run the algorithm increases linearly with the number of input items.
You may notice that in addition to examining each element once, there is a check to ensure that the array is not empty and a step that initializes the curMax variable. It may seem more accurate to call this an O(n + 2) function to reflect these extra operations. Big-O analysis, however, yields the asymptotic running time, the limit of the running time as n gets very large. As n approaches infinity, the difference between n and n + 2 is insignificant, so the constant term can be ignored. Similarly, for an algorithm running in n + n2 time, the difference between n2 and n + n2 is negligible for very large n. Thus, in big-O analysis, you eliminate all but the highest-order term, the term that is largest as n gets very large. In this case, n is the highest-order term. Therefore, the CompareToMax function is O(n).
The analysis of CompareToAll is a little more difficult. First, you have to make an assumption about where the largest number occurs in the array. For now, assume that the maximum element is at the end of the array. In this case, this function may compare each of n elements to n other elements. Thus, there are n(n examinations and this is an O(n2) algorithm.
The analysis so far has shown that CompareToMax is O(n) and CompareToAll is O(n2). This means that as the array grows, the number of comparisons in CompareToAll will become much larger than in CompareToMax. Consider an array with 30,000 elements. CompareToMax compares on the order of 30,000 elements, whereas CompareToAll compares on the order of 900,000,000 elements. You would expect CompareToMax to be much faster because it examines 30,000 times fewer elements. In fact, one benchmark timed CompareToMax at less than .01 seconds, while CompareToAll took 23.99 seconds.
The fastest-possible running time for any run-time analysis is O(1), commonly referred to as constant running time. An algorithm with constant running time always takes the same amount of time to execute, regardless of the input size.
Best, Average, and Worst Cases
You may think this comparison was stacked against CompareToAll because the maximum value was at the end. This is true, and it raises the important issues of the best case, average case, and worst case running times. The analysis of CompareToAll was a worst-case scenario: The maximum value was at the end of the array. Consider, however, the average case, where the largest value is in the middle. You end up checking only half the values n times because the maximum value is in the middle. This results in checking n (n / 2) = n2/ 2 times. This would appear to be an O(n2/ 2) running time. Consider, though, what the 1/2 factor means. The actual time to check each value is highly dependent on the machine instructions that the code translates to and then on the speed at which the CPU can execute the instructions. Therefore, the 1/2 doesn’t mean very much. You could even come up with an O(n2) algorithm that was faster than an O(n2/ 2) algorithm. In big-O analysis, you drop all constant factors, so the average case for CompareToAll is no better than the worst case. It is still O(n2).
The best-case running time for CompareToAll is better than O(n2). In this case, the maximum value is at the beginning of the array. The maximum value is compared to all other values only once, so the result is an O(n) running time.
Note that in CompareToMax, the best-case, average-case, and worst-case running times are identical. Regardless of the arrangement of the values in the array, the algorithm is always O(n).
Ask the interviewer which scenario they’re most interested in. Sometimes there are clues to this in the problem itself. Some sorting algorithms with terrible worst cases for unsorted data may nonetheless be well suited for a problem if the input is already sorted.
Optimizations and Big-O Analysis
Algorithm optimizations do not always yield the expected changes in their overall running times. Consider the following optimization to CompareToAll: Instead of comparing each number to every other number, compare each number only with the numbers that follow it in the array. In essence, every number before the current number has already been compared to the current number. Thus, the algorithm is still correct if you compare only to numbers occurring after the current number.
What’s the worst-case running time for this implementation? The first number is compared to n numbers, the second number to n – 1 numbers, the third number to n – 2, resulting in a number of comparisons equal to n + (n – 1) + (n – 2) + (n – 3) + … + 1. This is a very common result, a mathematical series whose answer is expressed as n2/2 + n/2. n2 is the highest-order term, so this version of the algorithm still has an O(n2) running time in the worst case. For large input values, the change you make to the algorithm has no real effect on its running time.
O(1)
O(1) describes an algorithm that will always execute at the same time (or space) regardless of the size of the input dataset.
bool IsFirstElementNull(IList<string> elements)
{
return elements[0] == null;
}
O(N)
O(N) describes an algorithm whose performance will grow linearly and in direct proportion to the size of the input dataset. The example below also demonstrates how Big O favors the worst-case performance scenario; a matching string could be found during any iteration of the for loop and the function would return early, but Big O notation will always assume the upper limit where the algorithm will perform the maximum number of iterations.
bool ContainsValue(IList<string> elements, string value)
{
foreach (var element in elements)
{
if (element == value) return true;
}
return false;
}
O(N2) represents an algorithm whose performance is directly proportional to the square of the size of the input dataset. This is common with algorithms that involve nested iterations over the data set. Deeper nested iterations will result in O(N3), O(N4) etc.
bool ContainsDuplicates(IList<string> elements)
{
for (var outer = 0; outer < elements.Count; outer++) {
for (var inner = 0; inner < elements.Count; inner++) {
// Don't compare with self
if (outer == inner) continue;
if (elements[outer] == elements[inner]) return true;
}
}
return false;
}
O(2N) denotes an algorithm whose growth doubles with each addition to the input data set. The growth curve of an O(2N) function is exponential - starting off very shallow, then rising meteorically. An example of an O(2N) function is the recursive calculation of Fibonacci numbers:
int Fibonacci(int number)
{
if (number <= 1) return number;
return Fibonacci(number - 2) + Fibonacci(number - 1);
}
Logarithms are slightly trickier to explain so I'll use a common example:
Binary search is a technique used to search sorted data sets. It works by selecting the middle element of the data set, essentially the median, and compares it against a target value. If the values match it will return success. If the target value is higher than the value of the probe element it will take the upper half of the data set and perform the same operation against it. Likewise, if the target value is lower than the value of the probe element it will perform the operation against the lower half. It will continue to have the data set with each iteration until the value has been found or until it can no longer split the data set.
This type of algorithm is described as O(log N). The iterative halving of data sets described in the binary search example produces a growth curve that peaks at the beginning and slowly flattens out as the size of the data sets increase e.g. an input data set containing 10 items takes one second to complete, a data set containing 100 items takes two seconds, and a data set containing 1000 items will take three seconds. Doubling the size of the input dataset has little effect on its growth as after a single iteration of the algorithm the data set will be halved and therefore on a par with an input data set half the size. This makes algorithms like binary search extremely efficient when dealing with large data sets.
Summary
Just remember following steps for theta or big O analysis: figure out what the input is and what n represents, after that express the number of operations the algorithm performs in terms of n, afterwards eliminate all but the highest-order terms and later on remove all constant factors, because CPU and memory speed difference is not important in algorithm speed and velocity analyze. How are you calculating the speed of your algorithms? Do you think that your code can be done faster?
Big-O and Theta (Θ) notations are part of asymptotic analysis, which is used in computer science to describe the efficiency and performance of algorithms. Understanding these concepts is crucial for analyzing how different algorithms will scale with increasing input sizes. Let’s break down what each of these terms means and how they differ:
Big-O Notation (O):
Big-O notation provides an upper bound of an algorithm's running time. It describes the worst-case scenario, showing the maximum amount of time an algorithm can possibly take to complete as a function of the input size, n. When we say an algorithm is O(f(n)), we mean that the running time grows no faster than a certain rate relative to the size of the input.
For example, if an algorithm is O(n²), then its execution time increases at most at a rate proportional to the square of the size of the input data. This doesn’t mean the algorithm always runs in exactly n² time; rather, n² represents the upper limit of its running time as n grows.
Theta Notation (Θ):
Theta notation provides a tight bound of an algorithm's running time. Unlike Big-O, which provides only an upper limit, Theta notation bounds the function from both above and below, which means it defines both the upper and lower limits of the running time of an algorithm. When we say an algorithm is Θ(f(n)), it means that the running time grows exactly at the rate of f(n) for large enough values of n.
For instance, if we have an algorithm with a running time that is Θ(n²), we mean that there exists a constant multiple of n² that the running time will never exceed for sufficiently large n, and similarly, the running time will also not fall below a different constant multiple of n².
Differences Between Big-O and Theta:
- Upper vs. Tight Bound: Big-O notation provides an upper bound for the running time (i.e., the worst-case scenario), meaning the actual running time could be less. Theta notation provides a tight bound, meaning the running time is both not more and not less than a certain rate for large inputs.
- Usage: Big-O is more commonly used in describing algorithm efficiency, as worst-case scenarios are often more critical in algorithm analysis. Theta is used when an algorithm's running time is known to be within a certain range (not too much more or less).
- Interpretation: If you know an algorithm is O(f(n)), you know that it won’t be slower than f(n) for sufficiently large n. If you know it’s Θ(f(n)), you have a precise characterization: it’s neither significantly slower nor significantly faster than f(n) for large n.
Example:
Consider a simple for loop that iterates n times, performing a constant-time operation each iteration. This algorithm's running time is Θ(n) because it grows linearly with the input size: not faster, not slower. It's also O(n), which agrees with the fact that Θ(n) is a subset of O(n) – but the O(n) description is less precise since it doesn't exclude faster growth rates.
Understanding these notations and their differences is vital for accurately describing and understanding the performance characteristics of algorithms, especially when comparing different approaches to solve a problem.
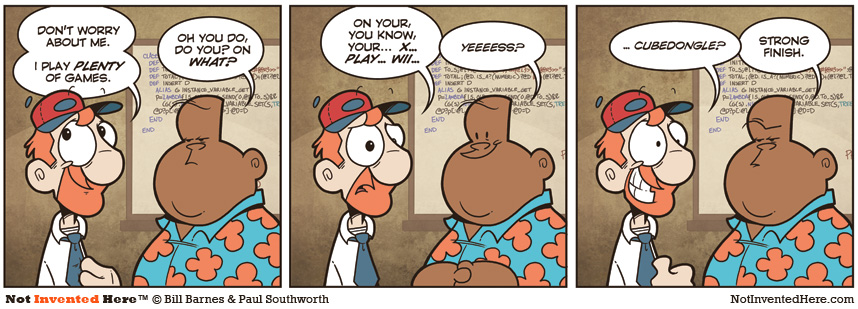
Best articles:NET Web frameworks, IOC pattern, IT articles, Visual Studio 2010 Tips.